2025: The Year Synthetic Data Goes Mainstream
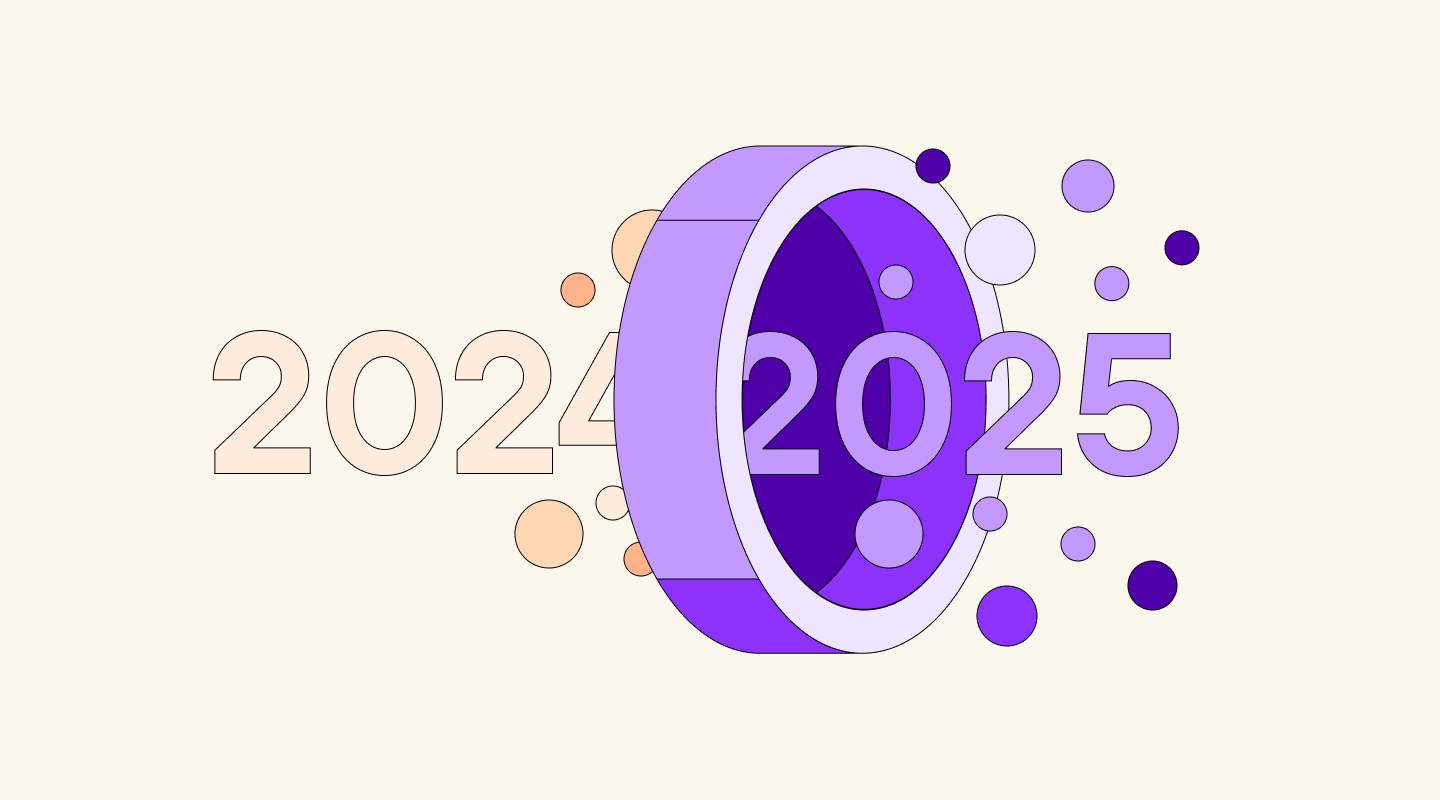
Happy New Year! As we kick off 2025, it’s clear that the enterprise AI landscape has undergone a massive shift. According to a recent Menlo Ventures report, AI spending in 2024 reached $13.8B—over 6x more than the previous year. This astounding growth reflects one core reality: AI isn’t just a research project or pilot anymore; it’s a critical driver of innovation across industries.
Here at Gretel, we’re excited to see more organizations moving from tinkering with AI to truly productizing it. But this rapid expansion has also surfaced new challenges. The same Menlo report indicates that 21% of AI pilots failed last year due to privacy concerns, and another 15% stalled because of complexity in model deployments. These statistics underscore a powerful lesson: success in enterprise AI is no longer just about having cutting-edge models—it’s about having high-quality, privacy-preserving data to fuel those models.
Where Enterprise AI is Today
- RAG Takes Center Stage
Retrieval-augmented generation (RAG) now powers 51% of enterprise AI implementations, a jump from 31% last year. Organizations are recognizing the need to ground AI outputs in trusted, contextual data rather than relying on generalized large language models alone. - Beyond Pilots: Scaling Up
While experimental pilots were the norm in 2023–2024, this year marks a turning point. AI is now in core production systems, from customer-facing chatbots to critical decision-support tools. - The Fine-Tuning Gap
Fine-tuning offers remarkable performance gains for domain-specific tasks, yet only 9% of enterprises are taking advantage. The primary barrier? High quality, diverse examples to provide for training- especially when privacy or regulatory constraints come into play. - Privacy Still a Pain Point
Privacy concerns caused 21% of failed AI projects last year—second only to implementation costs. In highly regulated sectors like healthcare and financial services, privacy hurdles have become a top priority. Collectively, these industries alone represent $600M of the enterprise AI market, indicating massive opportunities for solutions that address compliance requirements.
Why 2025 is the Year of Synthetic Data
As we engage with customers and partners at Gretel—from small teams to Fortune 500s—a common question emerges: “Where do we find or create the data we need to make my AI application work?” This is where synthetic data comes in.
1. Building Trust and Protecting Privacy
At its core, synthetic data enables you to generate datasets that look and behave like real-world data without revealing sensitive information. This lets teams:
- Prototype and Test Faster: Work with high-fidelity datasets while maintaining strong privacy protections.
- Share Data Securely: Collaborate across teams and geographies, even in regulated environments, without risking leaks of personal or proprietary information.
2. Customization for Domain-Specific Use Cases
Many companies realize their AI models need domain-specific data—whether it’s patient records or transcripts in healthcare or transaction logs and loan applications in finance. Synthetic data can be designed to include the nuances and edge cases your enterprise AI needs, leading to:
- Better Model Accuracy: Our recent benchmarks show that synthetic datasets tailored to specific use cases can outperform generic real-world datasets by as much as 25%.
- Accelerated Fine-Tuning: With the right training data, fine-tuned models- especially ones connected to RAG systems can offer unparalleled performance and efficiency.
3. Enhancing RAG Systems
RAG has emerged as the go-to architecture for many production systems because it pairs generative models with reliable data sources. Synthetic data can:
- Expand Your Knowledge Base: Generate “what if” scenarios or domain-specific documents that improve coverage and reduce hallucination risks.
- Enable Continuous Improvement: Quickly update or augment your knowledge base as you learn more about user behavior, market trends, or compliance rules.
Challenges to Watch For
Synthetic data isn’t a silver bullet. As more organizations adopt it:
- Quality Control
Not all synthetic data is created equal. Poorly generated data can lead to skewed or incomplete training that undermines model performance. Ensuring high fidelity and representativeness is paramount. - Complex Implementation
While synthetic data can accelerate development, it still requires robust tools and workflows to be integrated seamlessly into existing MLOps pipelines. Collaboration between data scientists, security teams, and domain experts is critical. - Regulatory Landscape
As regulators become more aware of synthetic data, new guidelines may emerge. We anticipate more scrutiny around how synthetic datasets are created and validated. For forward-thinking enterprises, this is an opportunity to lead by setting rigorous best practices.
How Gretel is Powering Enterprise AI Solutions
At Gretel, we’re committed to making synthetic data accessible and impactful for real-world enterprise AI applications. Throughout 2024, we introduced several features to help teams go from pilot to production faster:
- Data Designer: Our self-service platform that allows you to design high-quality synthetic datasets tailored to specific AI use cases.
- Safe Synthetic Data Generation: Differentially private training, PII replacement, and privacy evaluations built in, ensuring you can meet or exceed privacy and compliance requirements.
- MLOps Integrations: Native integrations with cloud AI services like AWS Bedrock, Azure AI OpenAI Fine-tuning, and Google BigQuery so your engineering and data science teams can integrate synthetic data in a familiar environment.
This year, we’re doubling down on advanced tooling for domain-specific data generation, as well as building out more robust reporting on data quality metrics. Ultimately, our goal is to ensure any team or enterprise can adopt synthetic data confidently, and see immediate ROI in their AI projects.
Looking Ahead: Opportunities in 2025
- Domain-Focused Solutions
In healthcare, finance, manufacturing, and beyond, synthetic data is set to unlock the next wave of AI use cases that were previously bottlenecked by data availability or compliance constraints. - Scaling Fine-Tuning
We anticipate a significant rise in fine-tuned models as synthetic data solves the shortage of specialized training examples. - Stronger Privacy Regulation
With more attention on data sovereignty, we expect an uptick in privacy-focused frameworks and legislation. This could further accelerate adoption of synthetic data, as it offers a secure path for AI development. - Rapid Prototyping and Testing
As RAG becomes ubiquitous, enterprises will demand test environments that quickly replicate real-world conditions. Synthetic data will play a key role in creating these sandbox environments for more robust, reliable AI systems.
Final Thoughts
2025 is the year synthetic data becomes a must-have in the AI toolkit. With spending ballooning and RAG systems dominating, enterprises can no longer rely on static, narrowly sourced datasets. High-quality synthetic data offers the agility, privacy, and specificity to solve the biggest enterprise AI challenges—from powering mission-critical applications to staying ahead of evolving regulations.
As a co-founder at Gretel, I’m excited about what’s coming. Our team is working tirelessly to develop tools and partnerships that make synthetic data practical and valuable for any AI-driven organization. This year will be all about scaling up, innovating responsibly, and ultimately helping you turn your AI ambitions into real business value.
Thank you for joining us on this journey. Here’s to a productive, privacy-safe, and mind-blowing 2025. Want to talk to our team about your own use cases for synthetic data? Find us on Discord, or reach out at hi@gretel.ai.
-Alex