Improve Machine Learning Performance with Synthetic Data
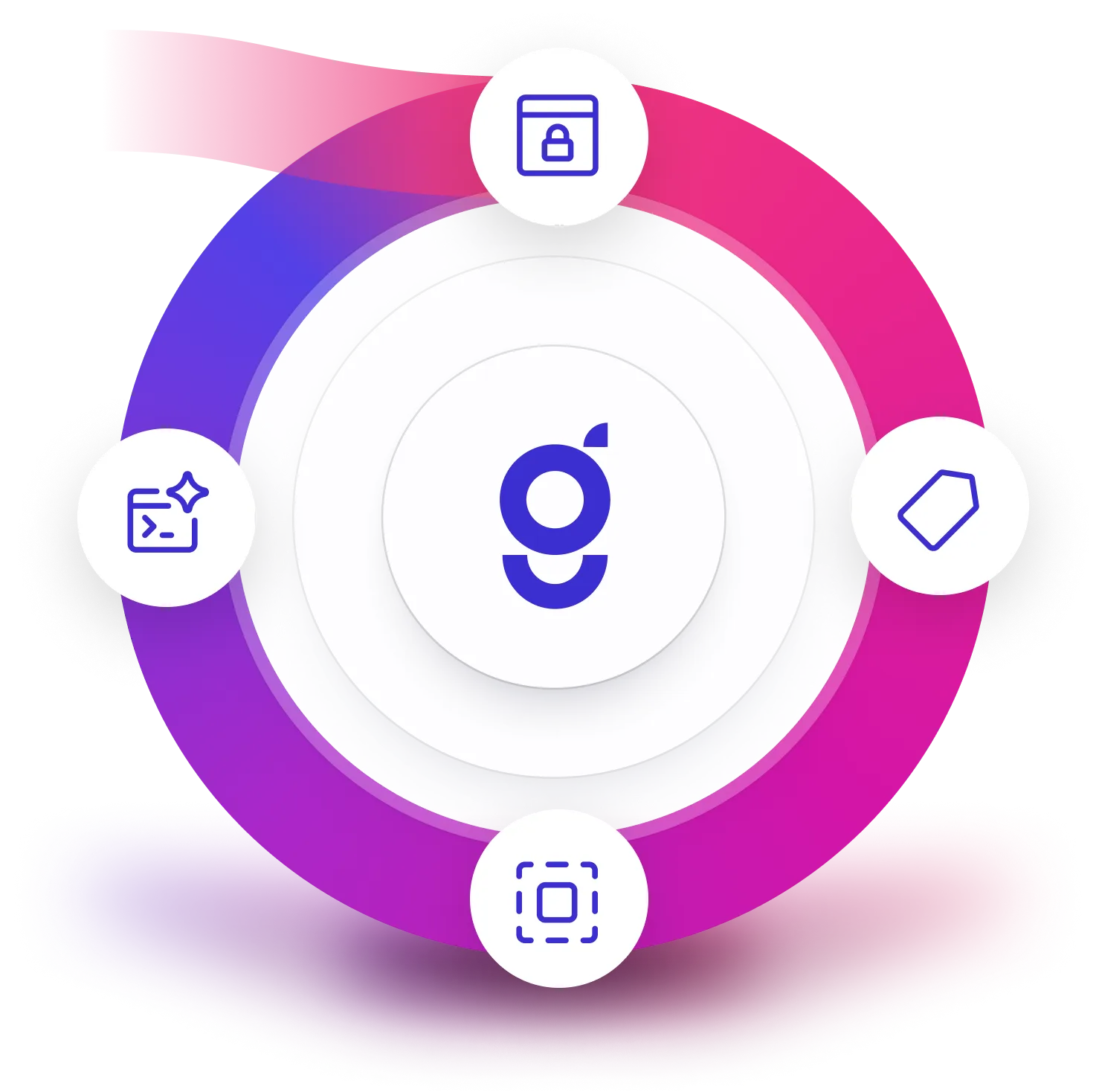
The ML training challenge
To unlock the value of machine learning models, organizations must train them on domain-specific proprietary data, enabling them to excel in specialized tasks. This is the most challenging task for machine learning teams. Referred to as the ‘data bottleneck’, the problem addresses the inability of organizations to rapidly extract value from AI due to challenges pertaining to training data availability, data quality, or data privacy. As a result, ML projects often fail to take flight, remain confined in innovation labs, and never reach production.
- Data Quality
Issues with data quality such as missing fields and unwanted bias greatly impact model performance, jeopardizing the utility of models in production.
- Data Availability
Training models requires large amounts of cleaned, curated, and annotated data. Collecting ground-truth data is time-consuming and expensive.
- Data Privacy
ML teams need access to sensitive data to train, evaluate, and improve models. Provisioning access to data takes months and raises compliance concerns.
Key Benefits
- Improve machine learning performance
Multiple synthetic data models purpose-built for producing high-quality and fully labeled data for more robust ML models.
- Faster time to value
Accelerate your most critical intelligent applications with on-demand access to synthetic training data that embeds directly in your ML pipelines.
- Safe training data for machine learning
Mathematically guaranteed privacy and mitigated risks of regulatory fines with provably private synthetic data.
Ready to try Gretel?
Get started in just a few clicks with a free account.
- Join the Synthetic Data Community
Join our Discord to connect with the Gretel team and engage with our community.
- Read our docs
Set up your environment and connect to our SDK.