Gretel Demo Day: Exploring the Future of Synthetic Data
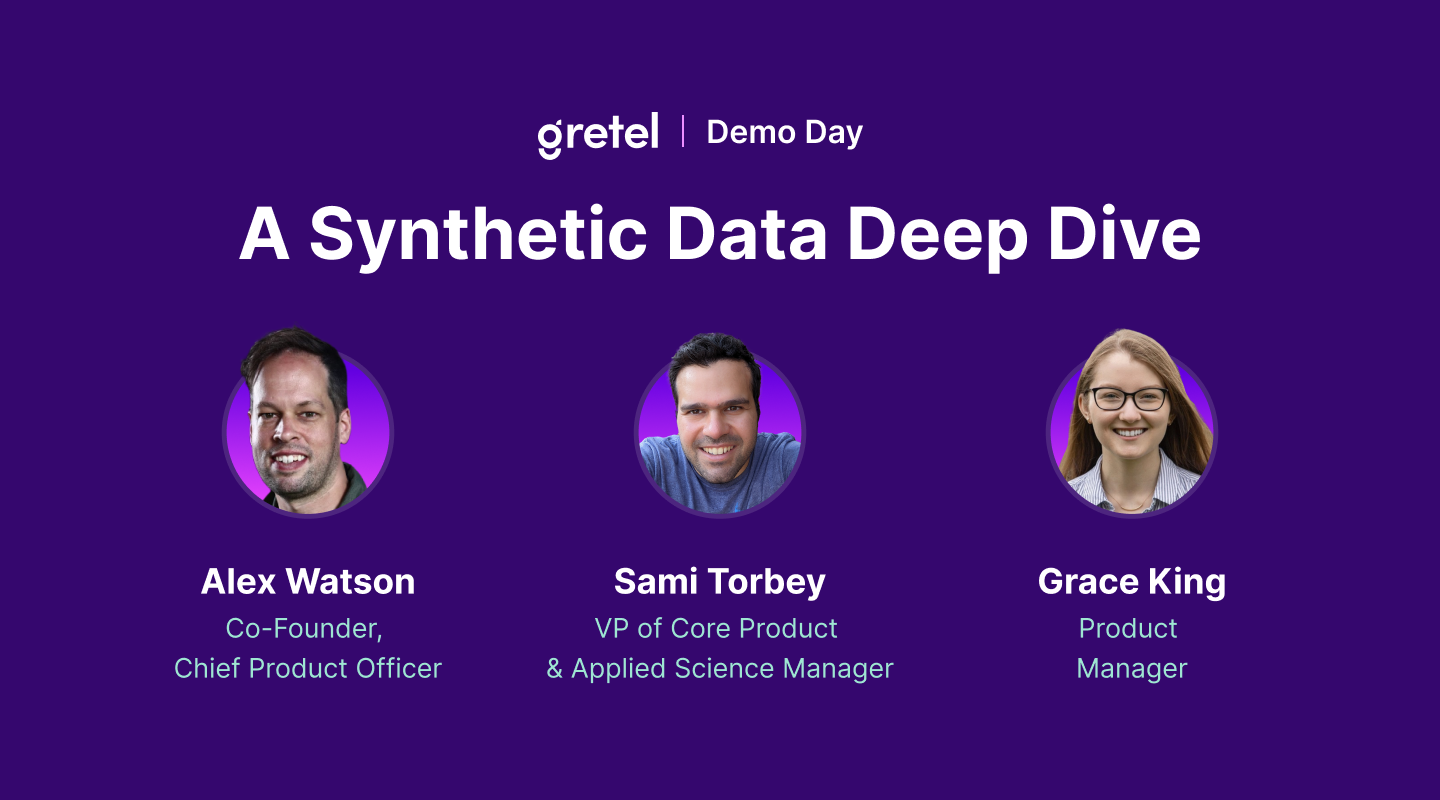
This week, we had a blast sharing some of our latest advancements with the AI community,Ā including our new Model Playground and Tabular LLM, a model tailored for tabular data generation. We also demoed our new no-code solutions for operationalizing and automating synthetic data generation tasks in your existing data pipelines. In case you missed it, below we cover key highlights from the Gretel Demo Day. Weāve included timestamped links throughout so you can follow along with the video recording. Letās go! šĀ
What Weāre Building
At Gretel we believe data is infinitely more valuable when it can be safely shared. Thatās why weāre focused on building better data that excels on downstream performance while safeguarding data sensitivity and protecting personal privacy. That's where the science of synthesizing data comes in.Ā
While itās still early days for generating high quality synthetic versions of raw data that you can trust for secure analytics and model training, it is an emerging market thatās gaining momentum fast. Every day, we have conversations with data scientists and AI engineers who are unlocking enormous business value by synthesizing data. Synthetic data is increasingly becoming both a catalyst for innovation as well as a tool for privacy compliance and model validation. As Microsoft researchers recently showed, synthetic data can even enable the development of smaller, yet highly efficient models that can achieve state-of-the-art performance. This streamlined, cost-effective, and privacy-compliant solution suggests a promising future for responsible AI development.Ā
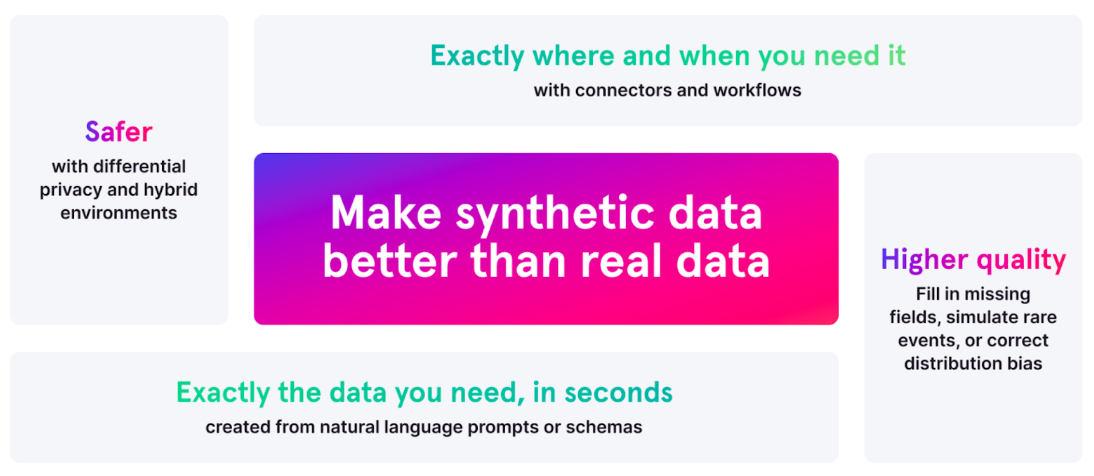
Policymakers are already leveraging synthetic data to enhance regulatory oversight, too. For instance, the UKās Financial Conduct Authority (FCA) used synthetic data to support its digital sandbox, which offered startups and enterprises a controlled regulatory environment for stress testing and evaluating their innovative financial technologies before deploying to market. Synthetic data was identified as the most valuable feature and resource by participants in the pilot program, and suggested that providing higher quality synthetic data will only enhance the benefits of the program. This underscores the growing importance and usefulness of synthetic data in innovative financial services environments, particularly in sandbox settings where new technologies and approaches are tested and refined.

In the U.S., President Bidenās Executive Order on Safe, Secure, and Trustworthy AI aimed at guiding responsible AI development and use across the federal government called for an acceleration in the federal adoption of privacy enhancing technologies, like synthetic data and differential privacy. The draft language of the EU's AI Act, particularly regarding model testing and training, recognizes synthetic data as separate but equivalent to anonymized data. If this language stays in the final law thatās expected to be enacted in 2024, it will have a transformative global impact on AI regulation and data governance.Ā
We expect these trends to continue with enterprise teams developing more bespoke, domain-specific models and applications, and policymakers and regulators adopting synthetic data to support privacy preservation without stifling innovation. Weāre building towards this future. Hereās how š
A Tabular LLM š¤
During the Demo Day, we showcased our new Tabular LLM - the first AI system designed for large-scale tabular data generation. The application excels at generating, augmenting, and editing datasets through natural language of SQL prompts. In one example, our VP of Product & Applied Science Sami Torbey, showed how you could augment or impute missing values, and even regionalize your dataset to be from France, complete with real French names and cities, at the click of a button. Cāest trĆØs chic! š® š«š·
Our co-founder and CPO Alex Watson walked through a more advanced example creating a highly accurate synthetic version of an HR dataset that mimicked the correlations and statistical properties of the real data.Ā ā
A Use Case for a Medical Researcher
To make this a bit more concrete, hereās how a prompt for a healthcare task might look, if you were doing clinical research and youāre data was biased due to limited records or historical inaccuracies:
āGenerate a diverse heart disease dataset with the following columns: first name, last name, age, gender, diagnosis, last doctor visit, blood pressure reading, weight. Balance male and female records in this dataset.āĀ
The model produces a table with name, age, gender, diagnosis, last doctor visit, blood pressure reading, and weight, as instructed. The male and female records in the table should conform to a 50/50 distribution. Results usually appear in 15 seconds or less, so if the results are not satisfactory, you can easily change or append to your previous prompt and try again.
A Use Case for a Financial Analyst
Imagine youāre a financial analyst at a hedge fund. Youāre tasked with forecasting e-commerce consumer trends in various regional markets. However, your current data is limited and missing a lot of context. With Gretelās Tabular LLM you could:Ā
- Use zero-shot prompting to bypass the cold start problem and generate comprehensive Consumer Packaged Goods (CPG) datasets from scratch, eliminating the necessity for pre-existing training data.
- Tailor your data for specific markets, such as generating a dataset exclusive to the Japanese market, complete with localized names, addresses, businesses, and language translation.
- Enhance your datasets by incorporating new elements, like adding an additional field for product reviews, improving the depth and value of the information available.
- Introduce more layers of relevant data using API documentation as your guide, creating a richer, more detailed dataset.
- Identify patterns, project user trends across various regions, and formulate data-driven strategies for market expansion, product investments, or user engagement.
Early access is now available, sign up to join the waitlist here.
A Model Playground š
Gretelās Model Playground is an interactive and engaging way for users to get tabular results from our proprietary LLM, especially if you have few or no training data (e.g. cold start). Secondarily, the playground offers a more natural and intuitive way for users to engage with our Gretel GPT model, which is powered by some of your favorite LLMs (e.g., Llama2 and Falcon). This ensures you get results back to help you tweak your prompt in near-real time, and also have a delightful experience while building data for your use case.
.gif)
Automated Workflows āļø
The main challenge we hear from enterprises is operationalizing synthetic data generation. Navigating the complexities of integrating this technology into existing workflows can be daunting.Thatās why weāve developed Gretel Workflows, our automated, end-to-end solution for simplifying synthetic data pipelines. Our Product Manager Grace King walks through a couple of ways you can easily add synthetic data generation to your tech stack.Ā Ā
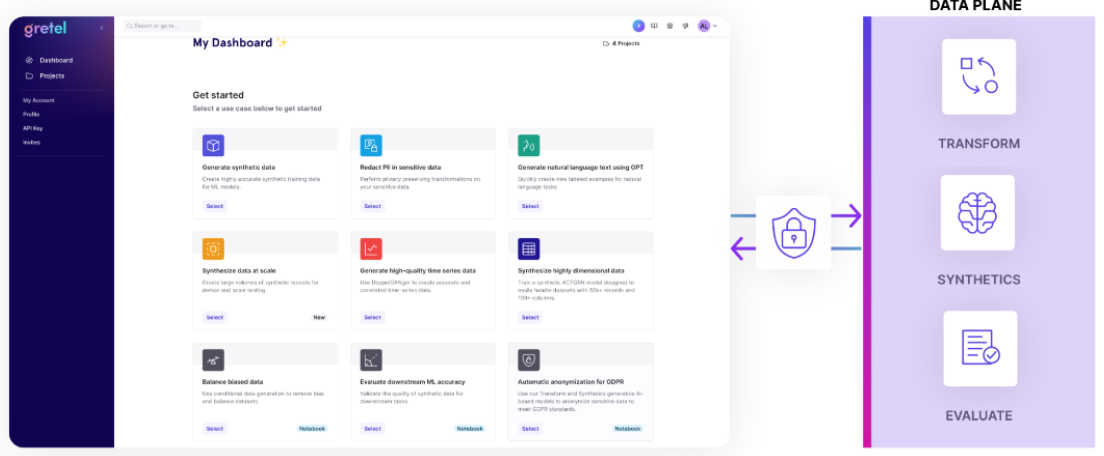
These workflows easily integrate it into your existing data pipelines using scheduling, cloud storage, database, and data warehouse connectors, and no-code configuration. This allows synthetic data to be created on-demand and made accessible wherever and whenever you need it.
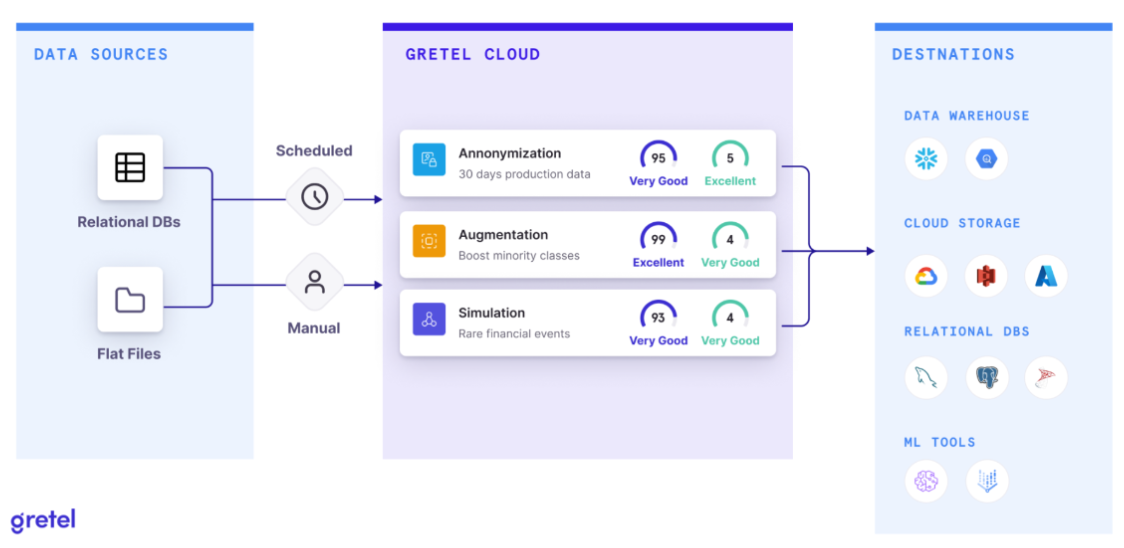
Data Quality Controls šļø
During the Demo Day, someone raised the important question of how we guarantee the synthetic data we generate is reliable and useful. The key lies in the quality of the models we design and use, and the data that feeds into them.
Think of it this way: If you're baking a cake, the quality of your ingredients and the recipe you follow will determine how well it turns out. Similarly, in creating synthetic data, the 'ingredients' (i.e., training data) we give to our AI models, and how we prompt the model with recipes of instructions and hyperparameter configurations, the better your synthetic data. To ensure we meet the highest standards of quality, Gretel provides a Synthetic Quality Score (SQS) for generated data ā itās like a taste test to assess if your synthetic data is accurate and private before you pull it out of the oven. šØš»āš³š„
The Future of Synthetics
2024 is shaping up to be another landmark year in the evolution of privacy-preserving generative AI. We are witnessing an increasing trend among clients, especially in healthcare, life sciences, and financial services, towards exploring multimodal synthetic data applications. There's a rising chorus from policymakers in the US, EU, and UK advocating for the development and implementation of privacy-enhancing technologies like synthetic data. Moreover, the tangible business value derived from these advancements, such as increased democratization of data access and custom AI model training, is becoming more evident every day. Our focus is on empowering engineers, researchers, and developers with intuitive, low-code tools to create clean, renewable, and privacy-preserving synthetic data. Quality data is the foundation for responsible AI development and data governance at scale.Ā
Weāre thrilled to be part of a growing community that includes privacy advocates and pioneers in data synthesis. Curious about Gretel or the world of synthetic data? Join the discussion in the Discord community channel or drop us a message at hi@gretel.ai.Ā