Thank you! Your submission has been received!
Oops! Something went wrong while submitting the form.
Gretel Blog
Learn more about Synthetic Data from Gretel experts – engineers, data scientists and our AI research team.
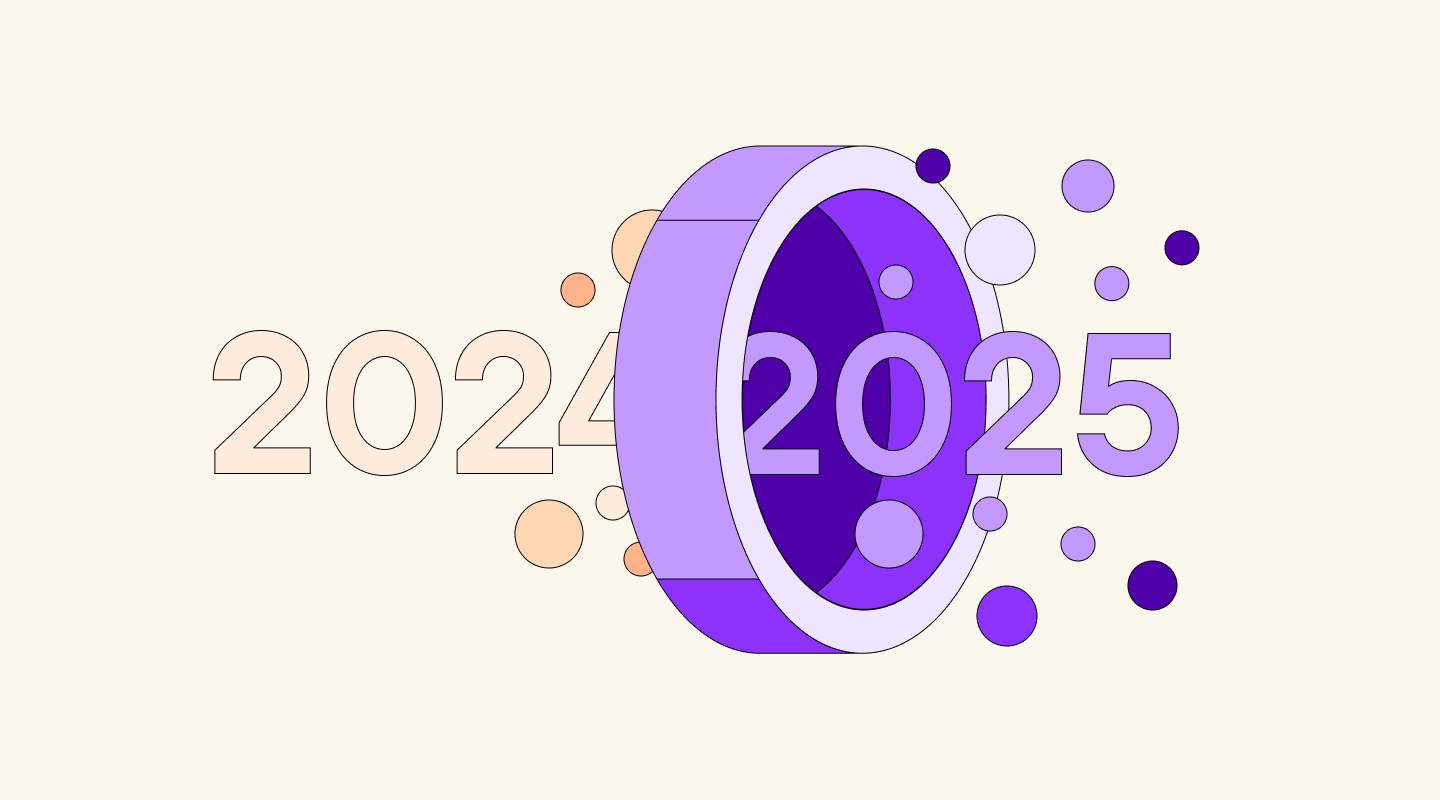
2025: The Year Synthetic Data Goes Mainstream
2025: The Year Synthetic Data Goes Mainstream
How synthetic data is transforming enterprise AI in 2025 by addressing privacy, fine-tuning, and scaling challenges.
Synthetics
Policy
RAG
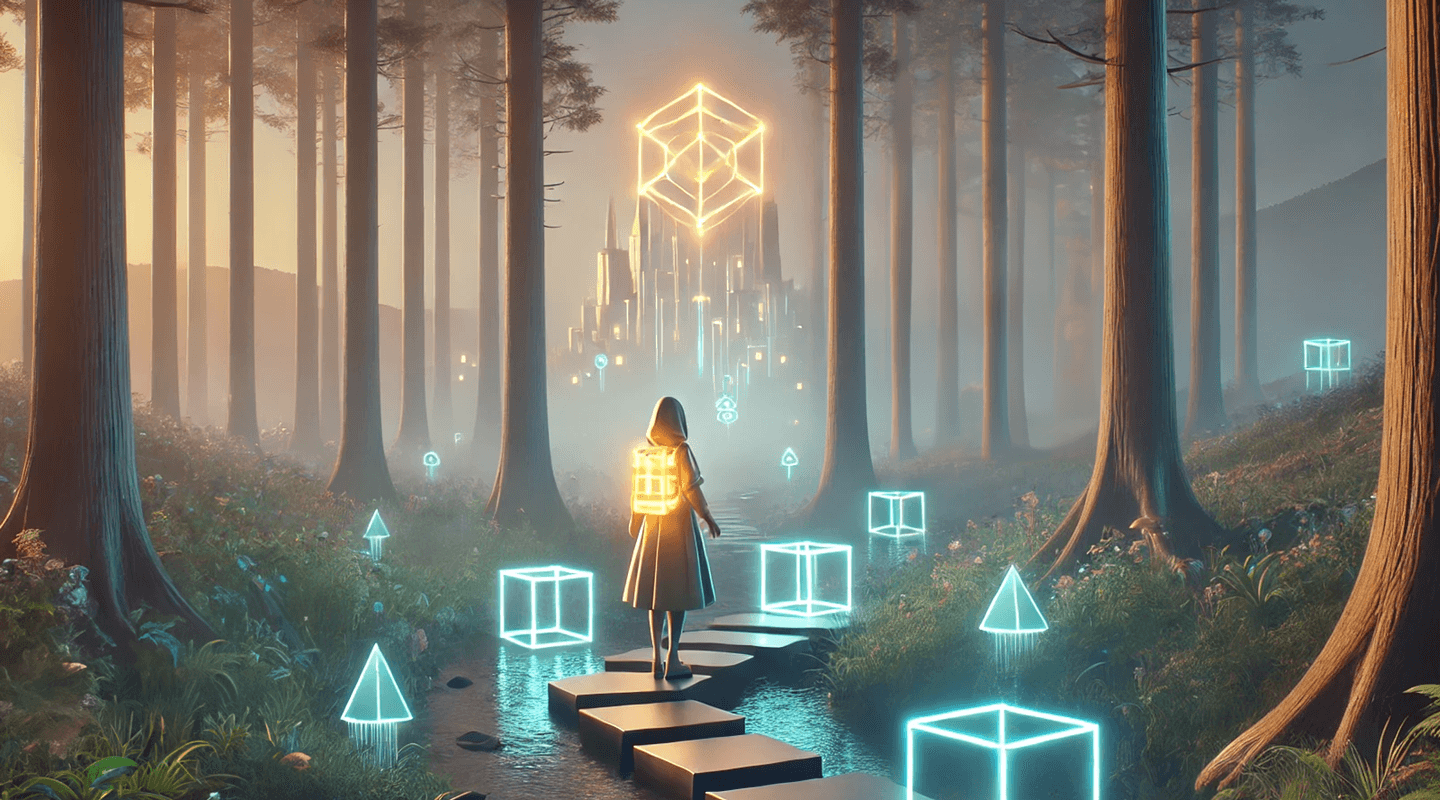
Building Datasets to Enable Safer AI Responses
Building Datasets to Enable Safer AI Responses
Gretel releases an open synthetic dataset to align language models for safety.
Gretel Open
Synthetics
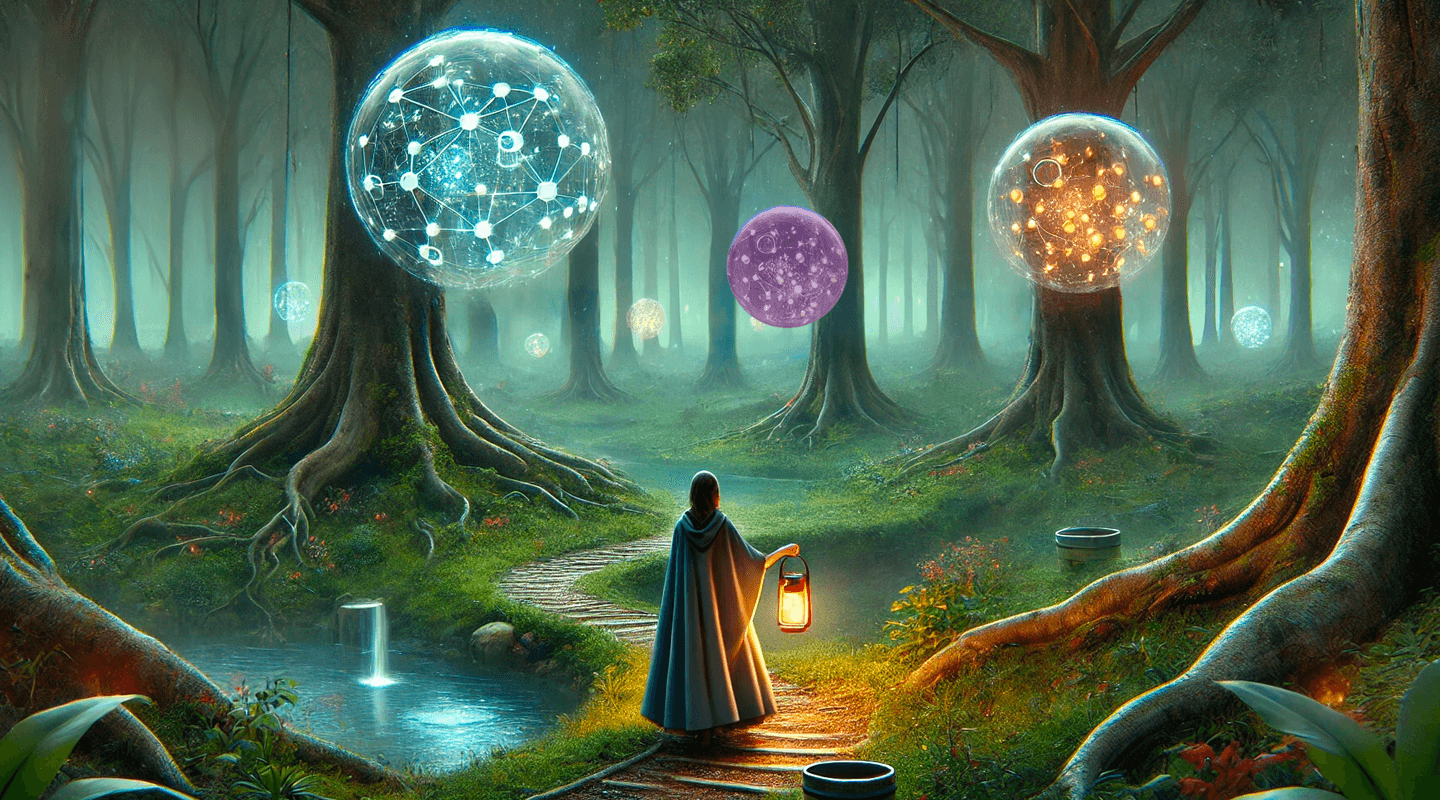
Introducing Model Suites for Synthetic Data Generation
Introducing Model Suites for Synthetic Data Generation
A new standard for ensuring regulatory compliance and managing the complexities of compound AI systems.
Synthetics
.png)
Teaching AI to control computers with Gretel Navigator on Amazon Bedrock
Teaching AI to control computers with Gretel Navigator on Amazon Bedrock
Use Gretel Navigator on Amazon Bedrock to create safe, scalable synthetic data for training AI to understand and execute tool commands.
Company News
Synthetics
Product updates
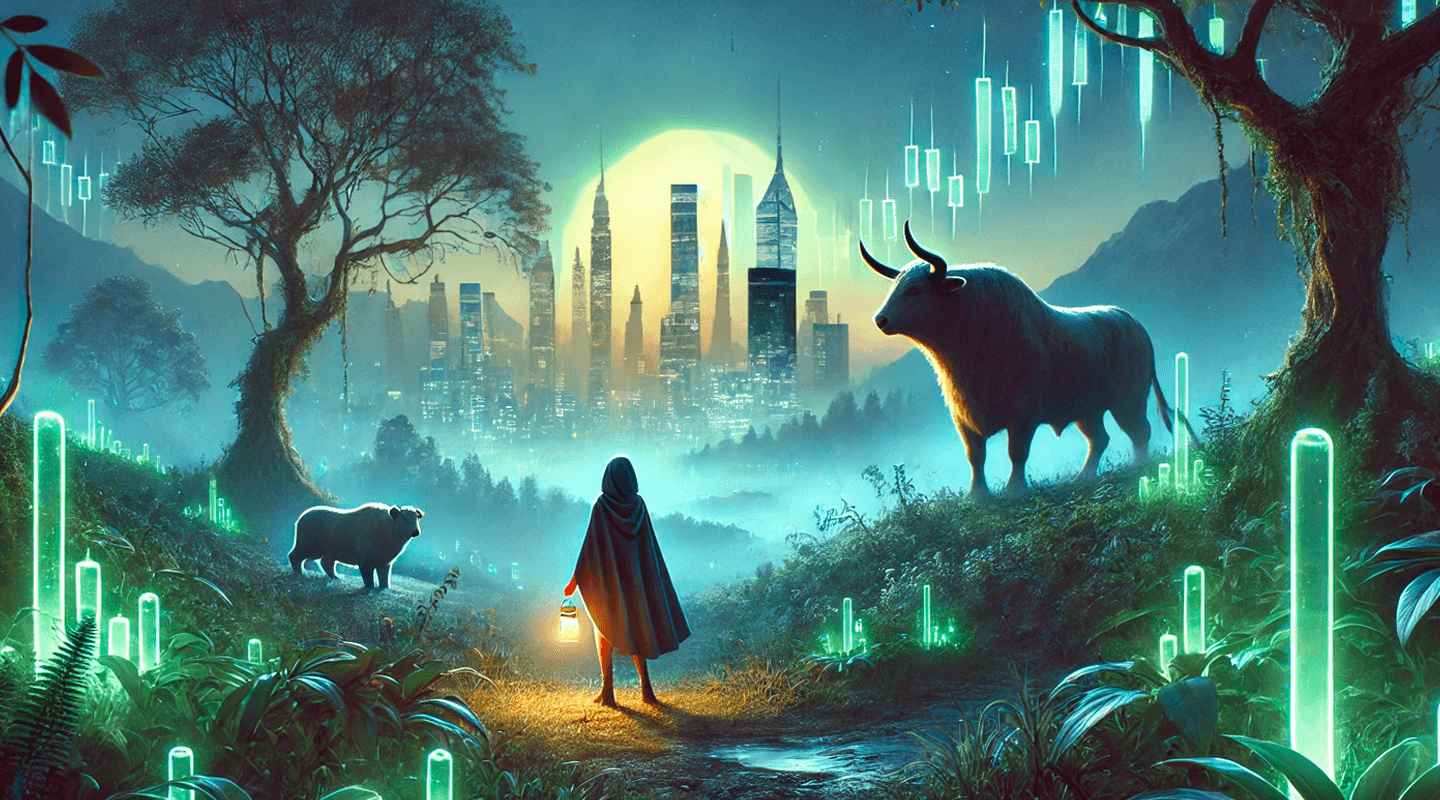
Accelerating FinTech Innovation with Natural Language to Code
Accelerating FinTech Innovation with Natural Language to Code
Train financial LLMs with Gretel's Synthetic Text-to-Python dataset to transform natural language into precise, domain-specific Python code for FinTech.
Gretel Open
Open Source
Synthetics
Finance
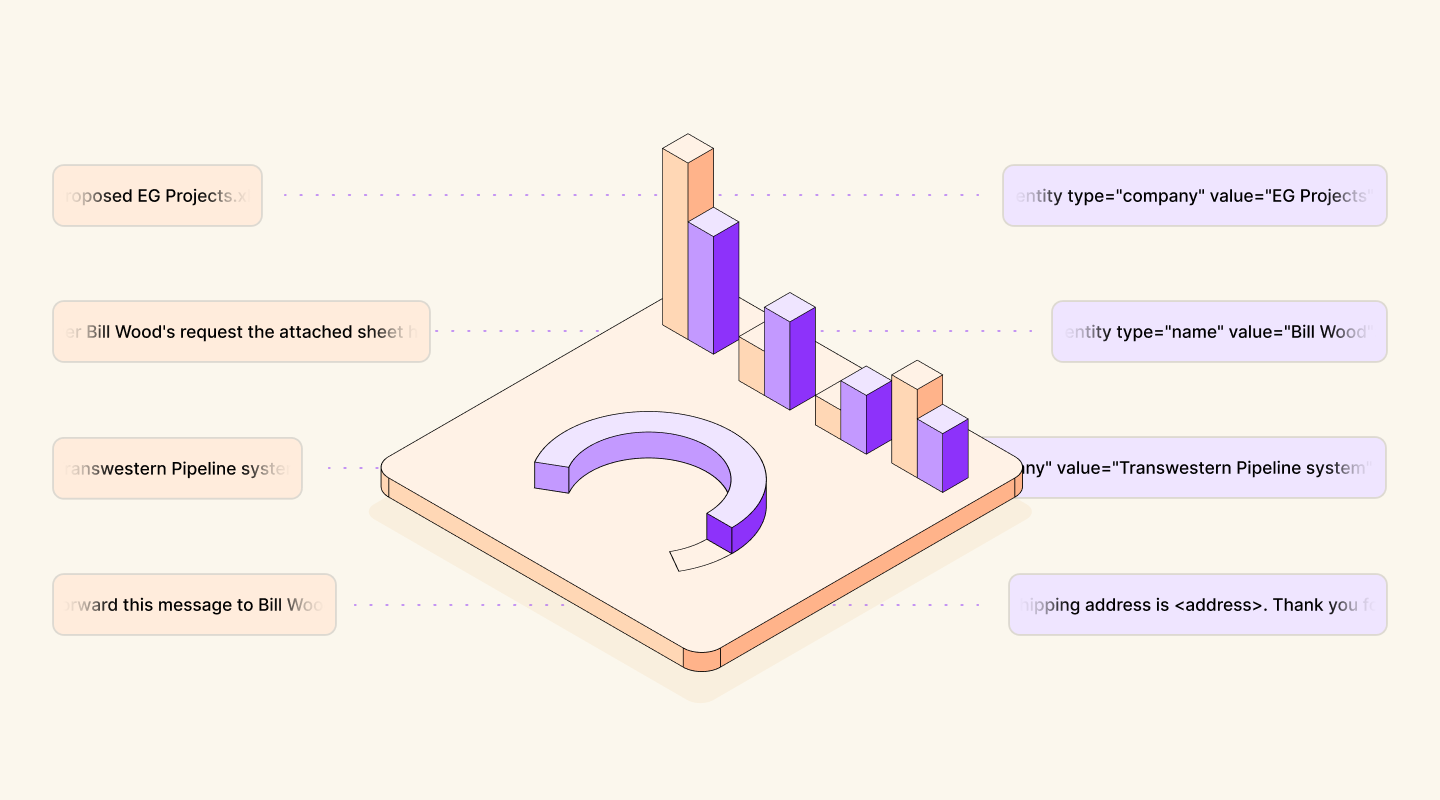
Quantifying PII Exposure in Synthetic Data
Quantifying PII Exposure in Synthetic Data
How to measure and minimize personally identifiable information (PII) risk in synthetic data.
Privacy
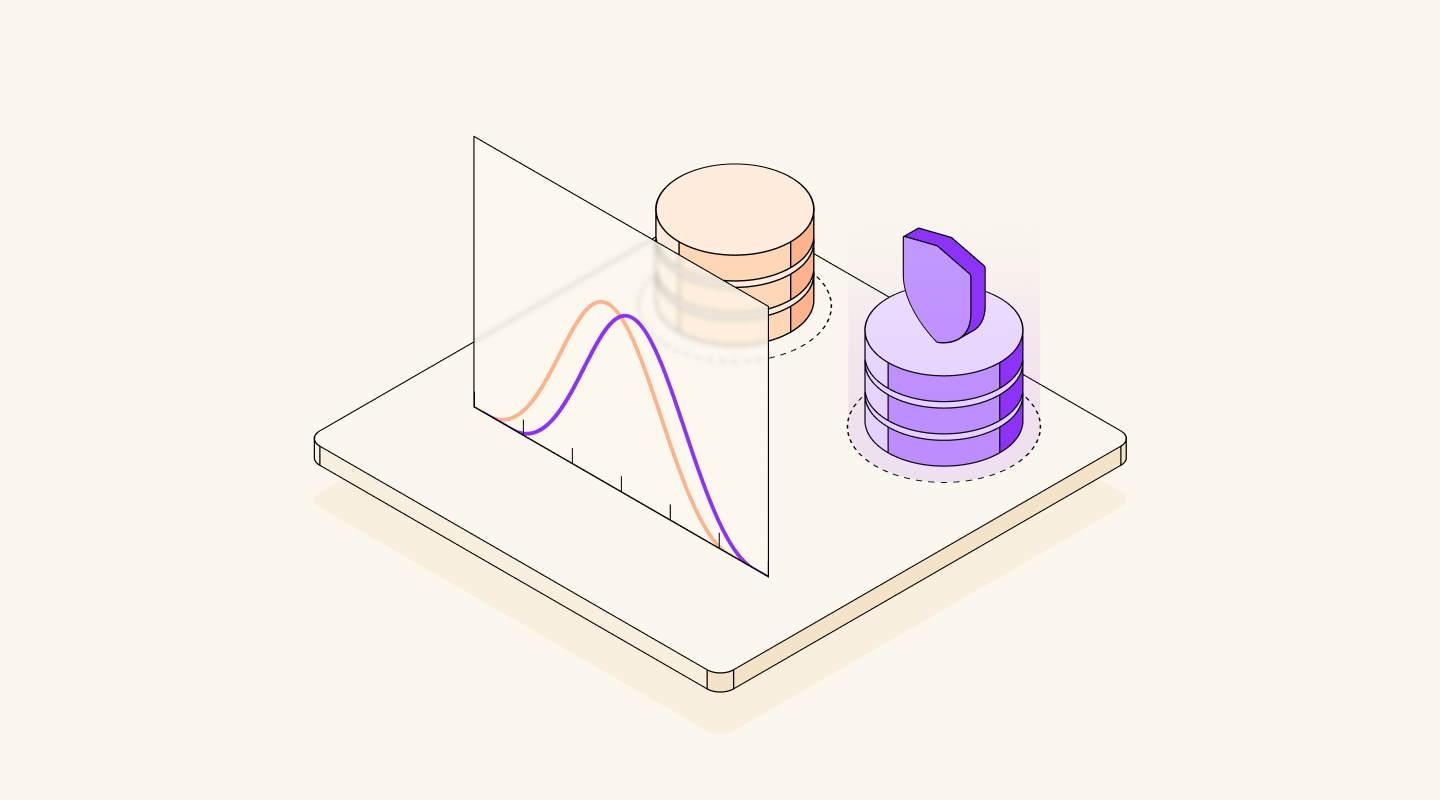
Generate Complex Synthetic Tabular Data with Navigator Fine Tuning + Differential Privacy
Generate Complex Synthetic Tabular Data with Navigator Fine Tuning + Differential Privacy
Learn how to generate secure, high-quality synthetic tabular data using Navigator Fine Tuning and Differential Privacy tools. 🛡️ 🚀
Differential Privacy
Fine Tuning
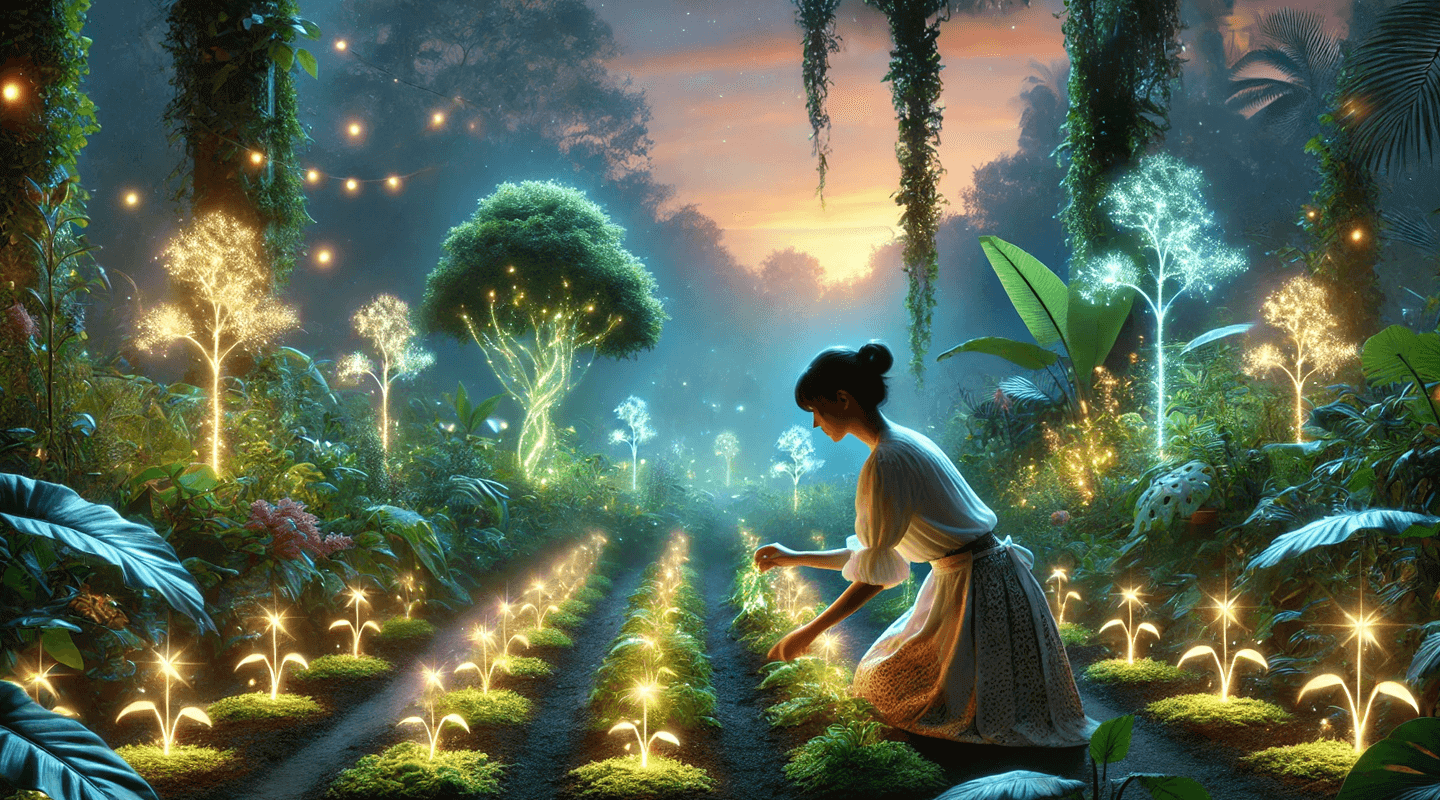
Sample-to-Dataset: Generate Rich Datasets from Limited Samples Using Data Designer
Sample-to-Dataset: Generate Rich Datasets from Limited Samples Using Data Designer
Seed to succeed: use the sample-to-dataset workflow to create diverse, large-scale synthetic datasets tailored to your needs with nothing but a few samples.
Synthetics
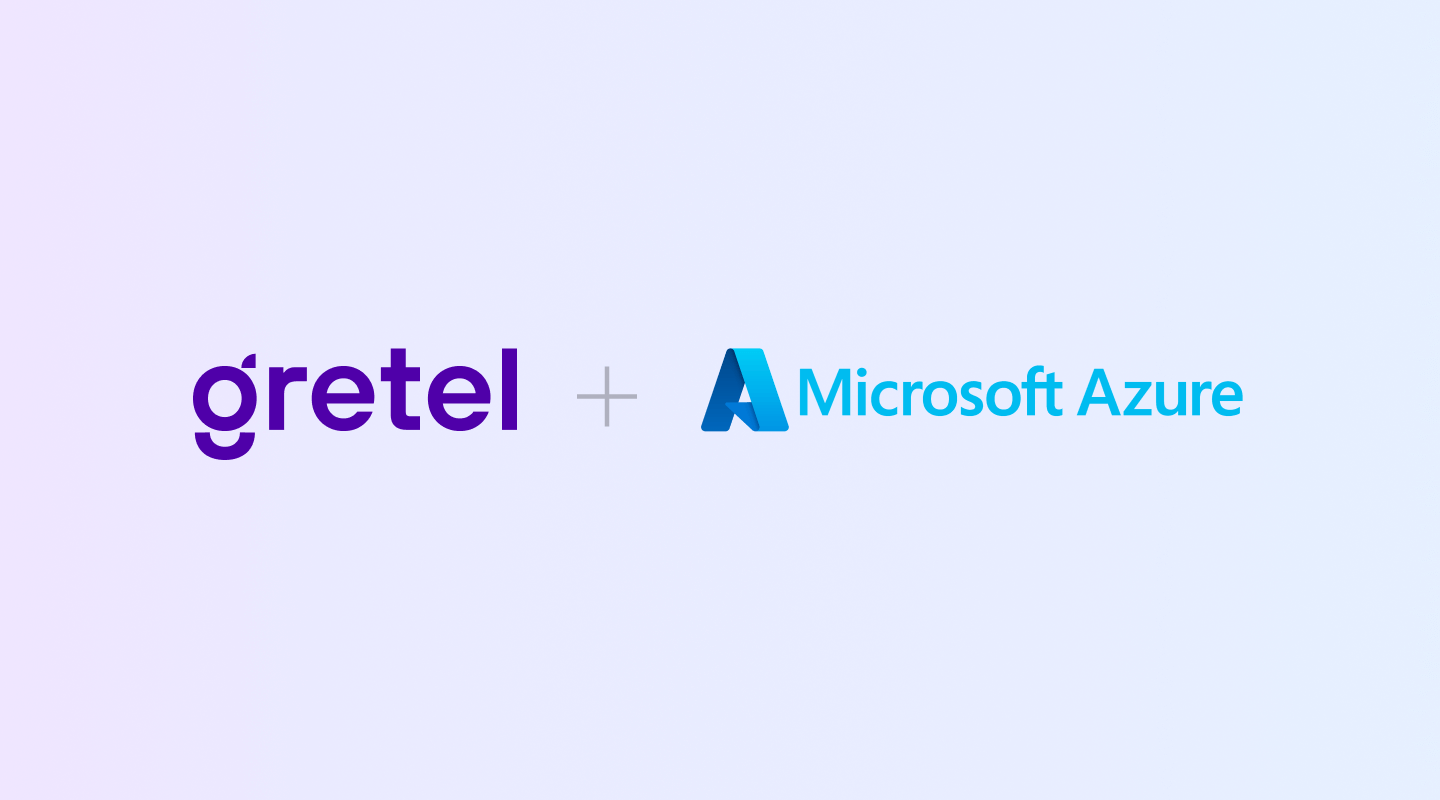
Privacy-preserving AI development with Azure & Gretel
Privacy-preserving AI development with Azure & Gretel
Leveraging Gretel's privacy-preserving synthetic data generation platform to fine-tune Azure OpenAI Service models in the financial domain.
Privacy
Finance
.png)
Build high-quality datasets for AI using Gretel Navigator Data Designer
Build high-quality datasets for AI using Gretel Navigator Data Designer
Gretel announces Data Designer for building high-quality, custom synthetic datasets with Navigator. Improve AI models on domain specific tasks and reasoning.
No items found.
.png)
GLiNER Models for PII Detection through Fine-Tuning on Gretel-Generated Synthetic Documents
GLiNER Models for PII Detection through Fine-Tuning on Gretel-Generated Synthetic Documents
Gretel fine-tuned, synthetically-enhanced GLiNER models for better PII & PHI detection—datasets included.
Gretel Open
Open Source
.png)
Fine-tuning Models for Healthcare via Differentially-Private Synthetic Text
Fine-tuning Models for Healthcare via Differentially-Private Synthetic Text
How to safely fine-tune LLMs on sensitive medical text for healthcare AI applications using Gretel and Amazon Bedrock
Synthetics
AI
Healthcare
Privacy
No results found.
Try a different search query, or select another topic.