What are the pros and cons of building versus buying?
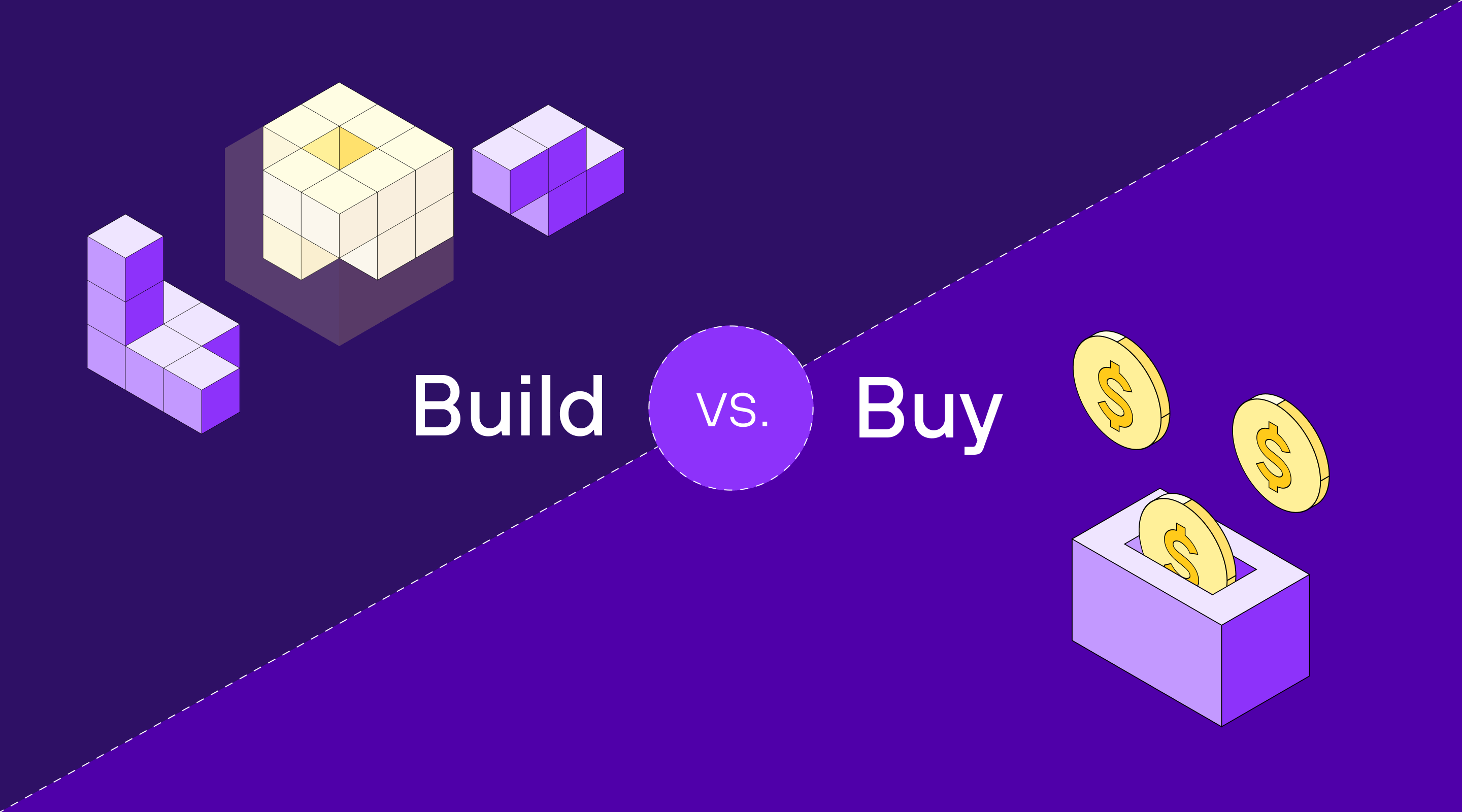
As synthetic data transforms the AI landscape, organizations face a pivotal investment decision: should they build a custom solution in-house or leverage a specialized platform? This choice involves more than features—it’s about allocating precious resources, whether in time, expertise, or capital. Building in-house isn’t a free lunch; it requires substantial investment in privacy and AI talent, and infrastructure to create a durable foundation.
With traditional anonymization methods proving insufficient for the genAI era, companies must quickly adopt advanced solutions to stay competitive while safeguarding security and scalability. The stakes are high: without a solid synthetic data strategy, early missteps can result in costly setbacks, leaving teams unprepared as AI advances.
Every organization has unique needs, so the optimal solution varies. For some, the control and flexibility of building in-house may appeal; for others, a platform’s efficiency and scalability are more compelling. This article explores both options, assessing challenges and benefits to guide you in making an informed decision that aligns with your organization’s goals and drives long-term AI success.
Complexity
Creating a versatile synthetic data solution entails far more than downloading the latest open-source models. Each data modality—tabular, text, or image—comes with unique technical hurdles. Developing, customizing, and scaling each type can quickly escalate costs and require specialized knowledge in machine learning, data engineering, and compliance.
A ready-made platform simplifies this complexity by offering a pre-configured toolkit for generating high-quality synthetic datasets across multiple data types. This solution frees up valuable time for teams, enabling them to focus on high-impact tasks, like optimizing machine learning pipelines and validating business outcomes, rather than navigating the complexities of integrating disparate models into a cohesive system.
Privacy & Security
Privacy protections are a non-negotiable component of any synthetic data strategy, yet achieving them in-house is one of the toughest challenges. Custom-built solutions must address a wide array of compliance requirements—ranging from differential privacy to anonymization—often without deep internal expertise. Even minor oversights can lead to costly regulatory misalignments, especially under GDPR, where non-compliance can incur expenses far surpassing initial development costs.
The stakes are heightened by the rising costs of data breaches. According to IBM’s Cost of a Data Breach 2024 report, the global average breach cost has reached $4.88 million, with even higher impacts in sectors like finance, where companies face average costs of $6.08 million.
A specialized synthetic data platform mitigates these risks by embedding established privacy frameworks and security protocols, simplifying compliance and providing peace of mind. It’s not just about meeting minimum standards; it’s about instilling trust at every step of the data generation process.
Data Quality
The true measure of any synthetic data solution is its performance in real-world applications. In-house builds that lack automated frameworks for measuring utility and quality may leave teams reliant on manual evaluations and static tests that don’t scale effectively. This creates a gap between the synthetic data’s theoretical performance and its practical value in deployment.
Automation is crucial to bridging this gap. Platforms often include built-in quality assurance tools that validate synthetic data against real-world tasks, ensuring performance meets expectations. In-house teams often underestimate the continuous effort required to monitor and uphold synthetic data quality, which can impede market trust and product transparency. Platforms, by contrast, offer dedicated layers of data risk management and evaluation metrics to address these needs, accelerating time to value while ensuring the data’s quality and reliability.
Scalability
Scaling an in-house synthetic data solution from proof-of-concept to enterprise-grade is where true complexity arises. Infrastructure demands grow as new data types and use cases are added, putting significant strain on internal resources to maintain both performance and compliance. Meeting these requirements calls for ongoing updates, rigorous testing, and frequent re-engineering.
Synthetic data solutions are often highly tailored to specific tasks, which means in-house teams may find it difficult to extend their solutions to new internal applications or clients. By contrast, platform solutions are designed with adaptability in mind, engineered to meet dynamic needs across different use cases. Opting for a scalable platform enables organizations to expand synthetic data capabilities without overextending their teams. Key factors, such as adaptable architecture, robust integrations, and hybrid deployment support, prevent the solution from becoming a bottleneck as business demands evolve.
Time to ValueÂ
In a fast-evolving field like AI, development delays can translate to missed opportunities. For many teams, the complexity of custom-building a synthetic data solution leads to extended timelines, causing critical milestones to be missed and project momentum to stall.
A ready-to-use platform, on the other hand, enables rapid deployment of synthetic data production, helping teams keep pace with innovation cycles and respond flexibly to new demands. This speed doesn’t just enhance project efficiency; it empowers teams to achieve quicker insights and concentrate on strategic decisions that drive business outcomes.
Choosing the Right Path
When deciding whether to build or buy, it’s essential to look beyond immediate costs and consider the full lifecycle of a synthetic data solution. Building in-house may provide maximum flexibility, but it also requires extensive resources and ongoing maintenance to remain effective. A specialized platform, by contrast, reduces complexity and risk, enabling teams to focus on innovation rather than foundational upkeep.
Ultimately, the right choice depends on where your organization wants to invest: in solving engineering challenges or accelerating AI success.
Ready to try Gretel?
Get started in just a few clicks with a free account.
- Join the Synthetic Data Community
Join our Discord to connect with the Gretel team and engage with our community.
- Read our docs
Set up your environment and connect to our SDK.